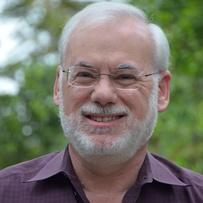
My research centers on the interplay between models and data, emphasizing decision making under uncertainty. I have been developing methods for modeling, simulation, analysis, and understanding of complex stochastic systems, as well as data-integration-based techniques for combining multiple simulation models to study complex systems of systems, e.g., involving population health and safety. My recent work on data-driven simulations uses machine learning methods to help facilitate the creation and deployment of simulation models for users who are not simulation experts. Other research has focused on techniques for efficiently representing, querying, and exploring large amounts of uncertain data, and using this data for prediction, optimization, and model adaptation in a seamless process. In other ongoing work, I am combining maximum entropy, machine learning, and data mining methods to estimate chronic disease prevalence in the presence of sparse data, and am using species-estimation methods to un-bias training data for machine learning models.